Downtime in metalworking is expensive. Lost production, missed deadlines, and the cost of repairs all add up quickly. Reactive maintenance – fixing things only after they break – is a costly and inefficient strategy.
Predictive maintenance, on the other hand, offers a proactive approach. It uses data analysis to anticipate equipment failures and schedule maintenance before they occur.
This blog post will explore how predictive maintenance can drastically reduce downtime in your metalworking operations.
Table of Contents
- The Downtime Problem in Metalworking
- How Predictive Maintenance Works
- Steps to Implement Predictive Maintenance
- Types of Data and Predictive Models Used
- Benefits and ROI of Predictive Maintenance
- Challenges and Considerations
- Resources for Virginia Metalworkers
- Conclusion and Next Steps
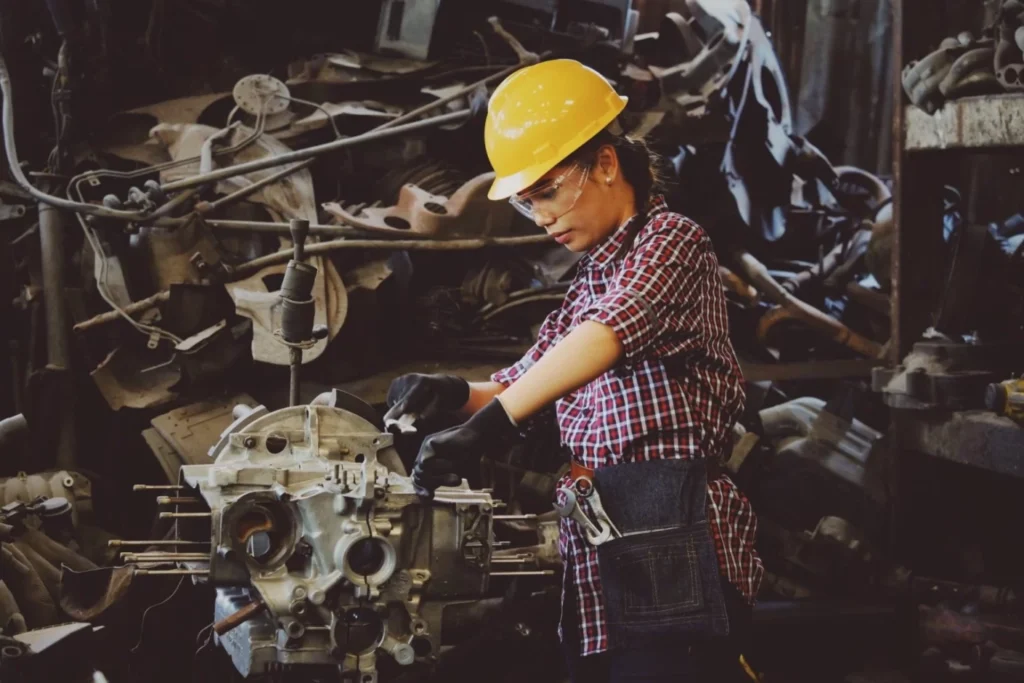
The Downtime Problem in Metalworking
The metalworking industry relies on sophisticated and often expensive machinery. A single machine breakdown can halt entire production lines, leading to major financial losses. According to an Information Technology Intelligence Consulting Research study, 98% of organizations report that a single hour of unplanned downtime costs over $100,000. For 33% of companies, that hourly cost exceeds $1 million.
With this data in mind, our GENEDGE experts predict that unplanned downtime can cost manufacturers an average of $50,000 per hour. This figure is even higher for complex metalworking processes.
The impact extends beyond immediate production losses; it can also affect:
- Delivery schedules
- Employee morale
- Product quality
- Safety
The Power of Predictive Maintenance
Predictive maintenance moves beyond scheduled maintenance (preventative maintenance) and reactive maintenance. Instead of following a fixed schedule or waiting for a failure, it uses data to predict when a component will likely fail. This allows for fast intervention, lowering downtime and extending the lifespan of equipment. Here’s how it works:
- Data Collection: Sensors are installed on machines to collect various data points, including vibration, temperature, pressure, and current.
- Data Analysis: Sophisticated software algorithms analyze this data to identify patterns and anomalies that indicate potential problems. Machine learning models can be trained to recognize specific failure signatures.
- Predictive Modeling: Based on the analysis, the software predicts the probability and timing of equipment failure.
- Maintenance Scheduling: This prediction helps businesses proactively plan and schedule maintenance activities, minimizing disruption to production.
Read more about technology and the impact of Industry 4.0 on the metalworking industry.
How To Introduce Predictive Maintenance to Your Metalworking Shop
1. Identify Critical Assets
Focus on the most critical machines that, if they fail, will cause the most downtime and financial losses. Prioritize those machines for sensor installation and data collection.
2. Sensor Selection and Installation
Choose sensors appropriate for the specific machines and the data you need to collect. Professional installation means everything for accurate data. Think about factors like the environment and the type of machinery. For example, high-temperature environments might need specialized sensors.
3. Data Acquisition and Storage
Invest in a data acquisition system capable of handling the volume of data generated by multiple sensors. Cloud-based solutions often offer scalability and easy access.
4) Software Selection
Choose predictive maintenance software compatible with your sensors and data acquisition system. The software should be capable of analyzing the data, generating predictions, and providing actionable insights. Many vendors offer industry-specific solutions for metalworking.
5) Training and Expertise
Your team needs training to effectively use the predictive maintenance software and interpret the data. Partner with a vendor or manufacturing quality consulting provider who can provide ongoing support.
Types of Data and Predictive Models Used
- Vibration Analysis: Detects imbalances, misalignment, bearing wear, and gear damage.
- Temperature Monitoring: Identifies overheating, lubrication problems, and electrical faults.
- Acoustic Emission: Detects cracks, leaks, and other structural defects.
- Current and Power Consumption: Indicates motor issues, overloaded circuits, and impending failures.
The predictive models used vary depending on the data and the specific application. Common techniques for metalworking technology include:
- Regression analysis: Predicts the remaining useful life (RUL) of a component based on historical data.
- Machine learning algorithms: Such as Support Vector Machines (SVMs) or Neural Networks, can learn complex patterns in the data and identify anomalies that might indicate impending failure. These are increasingly popular due to their ability to handle large datasets and complex relationships.
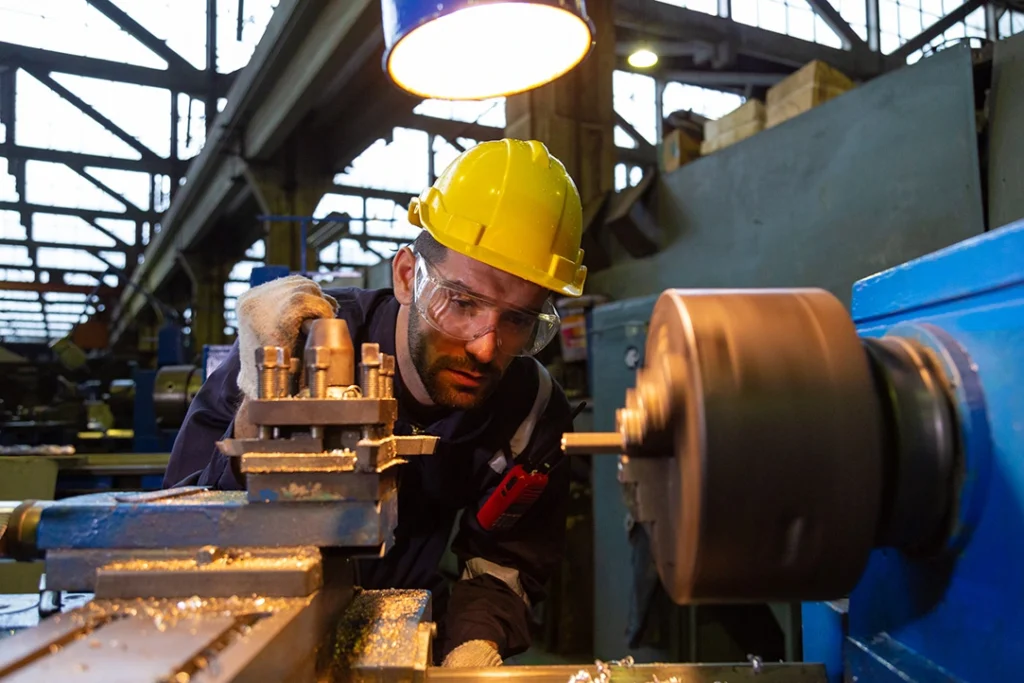
Benefits and ROI of Predictive Maintenance
- Proactive maintenance prevents unexpected breakdowns, minimizing production interruptions.
- Early detection of problems allows for timely repairs, extending the useful life of machinery.
- Predictive maintenance avoids unnecessary maintenance tasks and focuses resources on addressing actual problems.
- Early detection of potential hazards reduces the risk of accidents.
- Predictive maintenance allows for optimized inventory of spare parts.
Calculating the ROI of predictive maintenance requires careful consideration of the costs of implementation (sensors, software, training) and the savings from less downtime, maintenance costs, and improved equipment lifespan.
The formula:
ROI=Net Savings−CostsCosts×100
Manufacturing operations support from GENEDGE can help you track and measure ROI accurately.
Challenges and Considerations
- The upfront costs of sensors, software, and training can be high.
- Integrating data from multiple sources can be complex.
- Interpreting the data and making informed decisions requires skilled personnel.
- Predictive models can sometimes generate false alarms, leading to unnecessary maintenance.
Resources and Networks For Virginia Metalworkers
Virginia offers various resources that can assist metalworkers in adopting predictive maintenance:
- Virginia Economic Development Partnership (VEDP): The VEDP can provide information on grants, incentives, and other support programs related to technology adoption.
- Virginia Community Colleges: Many community colleges offer training programs in areas relevant to predictive maintenance, such as data analytics and industrial automation.
- Local Industry Associations: Networking with other Virginia metalworking businesses can facilitate knowledge sharing and adoption of best practices.
GENEDGE, with its focus on advanced manufacturing and metalworking technology adoption, can be a valuable resource for Virginia metalworkers implementing predictive maintenance and upgrading their manufacturing quality systems.
We offer expertise in identifying appropriate technologies, connecting businesses with relevant vendors, and providing training and support to help companies successfully integrate predictive maintenance solutions into their operations.
Boost your manufacturing’s efficiency by completing a No Cost Assessment today.